Facial Expression Recognition with Deep Learning
Motivation
Human emotion detection has a critical part in the interpersonal relationships. Emotions can generally be extracted from speech, hands and gestures of the body as well as through facial expressions. Being able to understand human emotions could improve the quality of the communications between human and machine. Furthermore, there is a wide range of industries that could benefit from emotion recognition such as healthcare, automotive, gaming, and many more.
While there are about 27 different human emotions, in this project, we plan to work with labeled data sets with seven distinct human emotions: happiness, sadness, fear, anger, surprise, disgust, and neutral. We aim to implement a two-step model: first, localize human faces in an image and second, recognize emotion expressed in those faces.
Part 1
Our first data set (’Dataset 1’), which is provided and maintained by Dataturks, has about 400 images with a bit more than 1000 faces. The data is available as a JSON file with 2 main components, an URL address of the image, and the face labels and bounding boxes of that image. We extracted those information from the JSON file, made an annotate function, and showed the images with the correct bounding boxes for the faces.
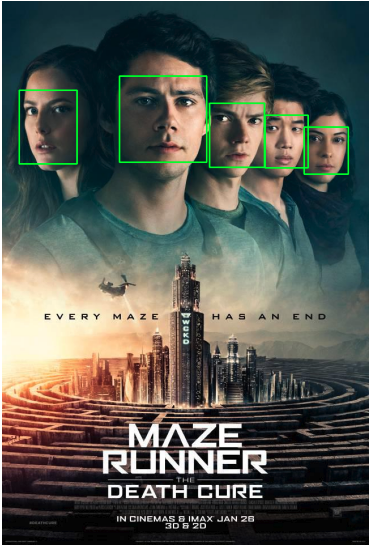
We selected a Faster R-CNN based model, the X101-FPN, available from Facebook’s Detectron2 package, Model Zoo for face detection task. Because this pre-trained model is developed for object recognition tasks with more than 30 different labels, we leverage the CNN layers for feature detections and then retrain the last few layers of the model using Dataset 1. The result is as follows:

Part 2
For facial expression recognition task, we used the FER2013 dataset (‘Dataset 2’). The data consists of 48x48 pixel grayscale images of human faces. The faces have been processed to be centered in the image and occupies about the same amount of space in each image. Each face is labeled as one of seven emotions.

We started with KNN and MLP as our baseline models with accuracies of 31.5% and 22.8% respectively. A random classifier would have 14.3% accuracy (7 labels) so the baseline models, even though having better accuracies, are still not good enough. This is where a CNN based model shines. We used 2 different CNN architectures, one simple CNN model with only 3 convolutional layers and a ResNet based CNN model. Both models were trained on the FER data set using Adam optimizer with learning rate of 0.001 and the loss is calculated using cross-entropy loss. The architecture of the two models are presented below:

The simple CNN model achieved a 54.8% accuracy, which already beat the 2 baseline models. The best accuracy we obtained is 62.8% from the ResNet based CNN model. The pre-trained ResNet model we are using as our initialization is trained on more than a million images from the ImageNet database. Therefore, it has already learned very good kernels in its convolutional layers and is able to extract useful features from the facial expression images without further training. That might be the reason why it outperforms our simple CNN model after the first training epoch.
Final thoughts
While the Faster R-CNN based model and the ResNet based CNN model achieved a very good result, there are instances where the models overdetect the bounding boxes or fail to capture the true emotions.
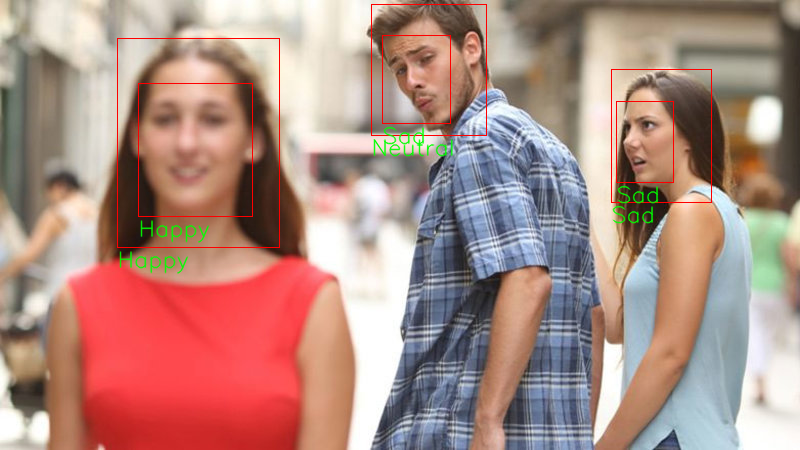
Facial expression recognition is a very challenging task, even for a human being. A trained human can be excellent at hiding true emotions and there could be no way to find out through facial expressions. Furthermore, a human face could show multiple expressions at the same time. With that said, machine learning is getting closer at matching human’s level, which could be exciting and terrifying at the same time.